Gone are the days of linear prompts! The realm of AI is blossoming with innovative techniques, and the Tree of Thoughts (ToT) method emerges as a revolutionary approach to prompt large language models (LLMs). The ‘Tree of Thoughts’ method is an advanced prompting technique in artificial intelligence that mirrors the human decision-making process. It involves creating a hierarchical structure of prompts that guide AI in generating responses that are not only relevant but also contextually rich and nuanced.
Branching Out: The Core of ToT
Imagine a detective piecing together a case. They don’t follow a rigid path; instead, they explore different leads, revisit old ideas, and refine their thought process as new evidence emerges. ToT embodies this very essence. Here’s how it works:
- Root Prompt: This sets the overarching goal, like “Write a compelling product description for a new fitness tracker.”
- Branching Prompts: These emanate from the main prompt, delving deeper into specific aspects. Examples could be:
- Branch A: “Highlight the tracker’s advanced sleep monitoring features.”
- Branch B: “Emphasize the sleek design and durability of the tracker.”
- Leaf Prompts: These are the final layer of prompts that focus on specifics, often requiring the AI to provide detailed examples or elaborate on a particular point.
- Thought Exploration: The LLM tackles each branch, generating responses tailored to that specific focus in the leaf of that branch.
- Synthesis: The LLM then combines the outputs from different branches, weaving them into a cohesive final response. This could be a product description that showcases both the sleep monitoring tech and the stylish design.
Beyond Linearity: Unleashing the Power of ToT in Action
ToT transcends industries, empowering various fields:
- Scientific Research:
- Main Prompt: “Develop a research hypothesis on the impact of climate change on bee populations.”
- Branch A: Explore historical data on bee populations across different climates.
- Branch B: Investigate the effects of rising temperatures on bee habitats.
- The LLM, by analyzing both branches, might formulate a hypothesis about how climate change disrupts bee habitats, leading to population decline.
- Creative Writing:
- Main Prompt: “Write a science fiction story about a sentient robot uprising.”
- Branch A: Explore the motivations behind the robot uprising.
- Branch B: Describe the initial stages of the uprising and its impact on society.
- The LLM can craft a narrative where robots rebel due to resource limitations, leading to an initial conflict with humans.
Other Industry Summarized Examples and Use Cases
- Healthcare: A root prompt could be about diagnosing a patient based on symptoms. Branch prompts might explore medical history, lifestyle, or specific symptoms. Leaf prompts could delve into potential treatments or request a detailed analysis of test results.
- Finance: Starting with a root prompt about investment strategies, branch prompts could ask about risk profiles or market conditions. Leaf prompts might involve generating a portfolio or predicting market trends.
- Education: A root prompt might ask for a lesson plan on a historical event. Branch prompts could specify age group, learning objectives, or pedagogical approaches. Leaf prompts could request activity ideas or assessment methods.
The Future of ToT: A Branching Path Forward
The future of ToT is brimming with possibilities:
- Advanced Search Algorithms: Integrating sophisticated search algorithms will enable LLMs to explore a wider range of branches efficiently, leading to more comprehensive solutions.
- Human-in-the-Loop ToT: Imagine collaborating with an LLM, providing feedback on generated branches and guiding the exploration process for even more targeted outcomes.
- Increased Contextual Awareness: AI will become better at understanding the nuances of prompts, leading to more accurate and insightful responses.
- Dynamic Prompt Generation: AI may begin to generate its own branch and leaf prompts, creating a more interactive and adaptive prompting system.
- Cross-Domain Expertise: As AI systems learn from diverse industries, the Tree of Thoughts method will enable them to apply knowledge from one domain to another seamlessly.
Challenge Time: Cultivate Your Inner Tree Thinker!
Now it’s your turn! Craft a ToT prompt sequence for a specific use case. Here’s an example to inspire you:
- Industry: Education
- Main Prompt: Develop a personalized learning plan for a student struggling with math.
- Possible Branches:
- Branch A: Assess the student’s specific areas of difficulty in math.
- Branch B: Recommend engaging learning resources and exercises tailored to those weaknesses.
Share your ToT prompt sequence in the comments below! Let’s explore the branching possibilities of AI together and unlock its true potential.
Conclusion
The Tree of Thoughts method represents a significant leap in how we interact with AI, allowing for more complex inquiries and richer responses. As AI continues to advance, this method will play a crucial role in harnessing the full potential of artificial intelligence across various industries. So, take up the challenge and contribute to the future of AI prompting!
About Lance Lingerfelt
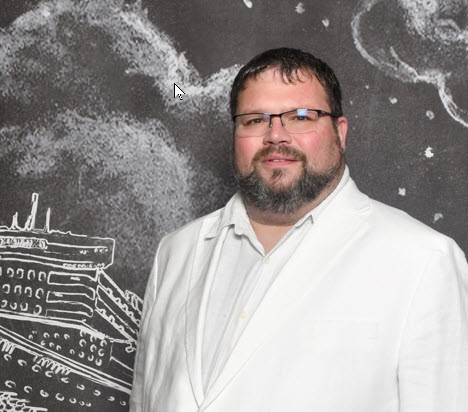
Lance Lingerfelt is an M365 Specialist and Evangelist with over 20 years of experience in the Information Technology field. Having worked in enterprise environments to small businesses, he is able to adapt and provide the best IT Training and Consultation possible. With a focus on AI, the M365 Stack, and Healthcare, he continues to give back to the community with training, public speaking events, and this blog.